Introduction
This course has been developed recently (Summer 2023). We welcome any feedback at help@pvactools.org or by submission of GitHub issues.
Motivation
Identification of neoantigens is a critical step in predicting response to checkpoint blockade therapy and design of personalized cancer vaccines.
This is a cross-disciplinary challenge, which involves genomics, proteomics, immunology, and computational approaches. We have built a computational
framework called pVACtools that, when paired with a well-established genomics pipeline, produces an end-to-end solution for neoantigen characterization.
pVACtools supports identification of altered peptides from different mechanisms, including point mutations, in-frame and frameshift insertions and deletions,
and gene fusions. Prediction of peptide:MHC binding is accomplished by supporting an ensemble of MHC Class I and II binding algorithms within a framework
designed to facilitate the incorporation of additional algorithms. Prioritization of predicted peptides occurs by integrating diverse data, including mutant
allele expression, peptide binding affinities, and determination of whether a mutation is clonal or subclonal. Interactive visualization via a Web interface allows
users to efficiently generate, review, and interpret results, selecting candidate peptides for individual experiments or patient vaccine designs. Additional modules
support design choices needed for competing vaccine delivery approaches. One such module optimizes peptide ordering to minimize junctional epitopes in DNA vector
vaccines. Downstream analysis commands for synthetic long peptide vaccines are available to assess candidates for factors that influence peptide synthesis. All
of the aforementioned steps are executed via a modular workflow consisting of tools for neoantigen prediction from somatic alterations (pVACseq, pVACfuse, and pVACbind),
prioritization, and selection using a graphical Web-based interface (pVACview), and design of DNA vector–based vaccines (pVACvector) and synthetic long peptide
vaccines. pVACtools is available at http://www.pvactools.org.
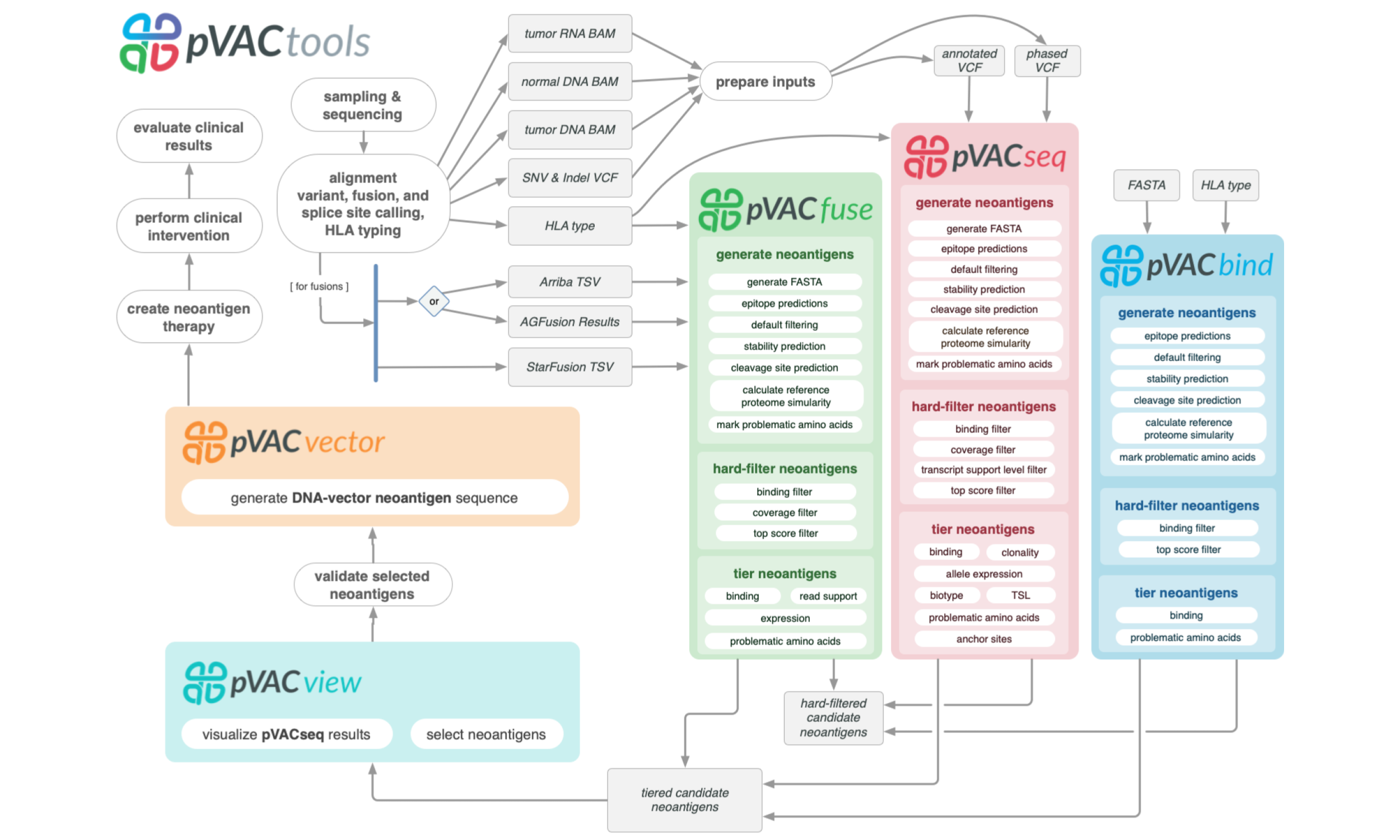
Background
Neoantigens are unique peptide sequences generated from mutations acquired somatically in tumor cells. These antigens provide an avenue for tumor-specific immune
cell recognition and have been found to be important targets for cancer immunotherapies (Keskin et al. 2018; Ott et al. 2017; Hilf et al. 2018). Effective neoantigens, presented by the
major histocompatibility complex (MHC) and thus introduced to the patient’s immune system, can prime and activate CD8+ and CD4+ T cells for downstream signaling of
cell-death. Patients with high tumor mutation burden tend to have stronger responses to neoantigen based immunotherapy treatments (Brown et al. 2014; Rizvi et al. 2015; Schumacher and Schreiber 2015).
DNA and RNA sequencing technologies allow researchers and clinicians to computationally predict potential neoantigens based on tumor-specific mutations.
However, neoantigen generation and presentation is complex, and a host of factors must be evaluated by complex analyses to characterize each potential neoantigen.
These include but are not limited to: somatic variant identification, tumor clonality assessment, RNA expression estimation, mRNA isoform selection, inference of
translated tumor specific peptides that arise from the somatic variant, and prediction of peptide processing, peptide transportation, peptide-MHC binding, peptide-MHC
stability and recognition by cytotoxic T cells (Richters et al. 2019).
pVACtools can be used as the final step in a well-established variant calling pipeline. It leverages existing tools with functionality related to variant annotation
(Ensembl VEP (McLaren et al. 2016)), identifying neoantigens from specific sources (e.g. fusions via star-fusion (Haas et al. 2019), AGFusion (Murphy and Elemento 2016), and Arriba (Uhrig et al. 2021)),
HLA typing (OptiType (Szolek et al. 2014), PHLAT (Bai, Wang, and Fury 2018)), peptide-MHC binding prediction (IEDB (Vita et al. 2018), NetMHCpan (Jurtz et al. 2017), MHCflurry (ODonnell et al. 2018),
MHCnuggets (Shao et al. 2020)), peptide-MHC stability (NetMHCstabpan (Rasmussen et al. 2016)], peptide processing (NetChop (Nielsen et al. 2005)), manufacturability
metrics (vaxrank (Rubinsteyn et al. 2017)), and reference proteome similarity (BLAST (Altschul et al. 1990)). Each of these tools tackles specific tasks within the broader goal of
antigen analysis and is utilized by pVACtools to provide an end-to-end integration of novel algorithms and established tools needed to discover, characterize, prioritize,
and utilize tumor-specific neoantigens in basic research and clinical applications. Combining pVACtools with existing variant calling pipelines provides an end-to-end
solution for neoantigen prediction and characterization.
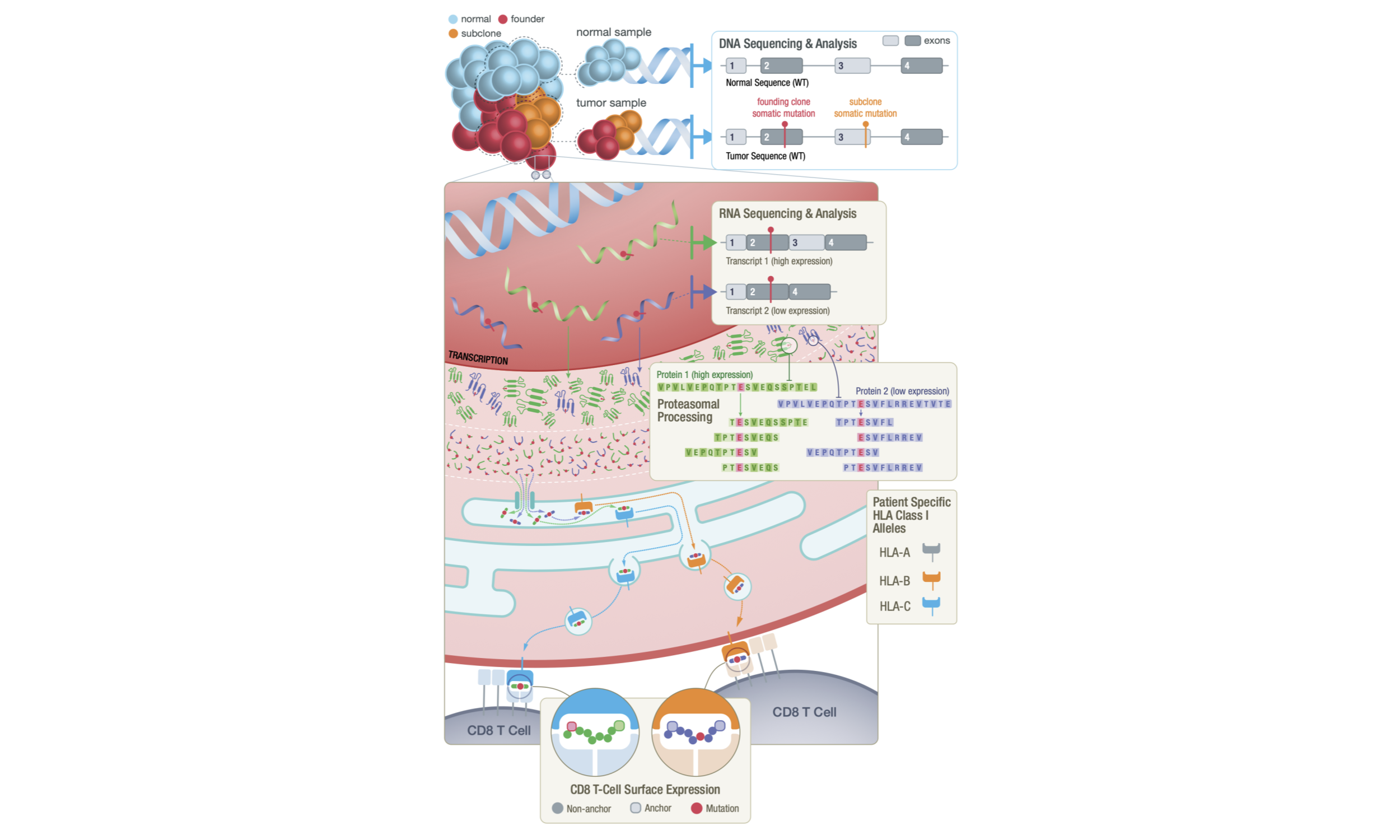
Target Audience
The course is intended for anyone seeking a better understanding of current best practices in neoantigen identification and prioritization using pVACtools.
It assumes that the learner is familiar with basic biology, genetics and immunology concepts.
Curriculum
This course will teach learners to:
- Understand key concepts of immunogenomics and neoantigen identification
- How to run the pVACtools software suite
- How to visualize and prioritize neoantigen candidates with pVACview
For more background on pVACtools please consult the core pVAC papers (Hundal et al. 2016, 2018, 2020).
Altschul, Stephen F., Warren Gish, Webb Miller, Eugene W. Myers, and David J. Lipman. 1990.
“Basic Local Alignment Search Tool.” Journal of Molecular Biology 215 (3): 403–10.
https://doi.org/10.1016/s0022-2836(05)80360-2.
Bai, Yu, David Wang, and Wen Fury. 2018.
“PHLAT: Inference of High-Resolution HLA Types from RNA and Whole Exome Sequencing.” In
Methods in Molecular Biology, 193–201. Springer New York.
https://doi.org/10.1007/978-1-4939-8546-3_13.
Brown, Scott D., Rene L. Warren, Ewan A. Gibb, Spencer D. Martin, John J. Spinelli, Brad H. Nelson, and Robert A. Holt. 2014.
“Neo-Antigens Predicted by Tumor Genome Meta-Analysis Correlate with Increased Patient Survival.” Genome Research 24 (5): 743–50.
https://doi.org/10.1101/gr.165985.113.
Haas, Brian J., Alexander Dobin, Bo Li, Nicolas Stransky, Nathalie Pochet, and Aviv Regev. 2019.
“Accuracy Assessment of Fusion Transcript Detection via Read-Mapping and de Novo Fusion Transcript Assembly-Based Methods.” Genome Biology 20 (1).
https://doi.org/10.1186/s13059-019-1842-9.
Hilf, Norbert, Sabrina Kuttruff-Coqui, Katrin Frenzel, Valesca Bukur, Stefan Stevanović, Cécile Gouttefangeas, Michael Platten, et al. 2018.
“Actively Personalized Vaccination Trial for Newly Diagnosed Glioblastoma.” Nature 565 (7738): 240–45.
https://doi.org/10.1038/s41586-018-0810-y.
Hundal, Jasreet, Beatriz M. Carreno, Allegra A. Petti, Gerald P. Linette, Obi L. Griffith, Elaine R. Mardis, and Malachi Griffith. 2016.
“pVAC-Seq: A Genome-Guided in Silico Approach to Identifying Tumor Neoantigens.” Genome Medicine 8 (1).
https://doi.org/10.1186/s13073-016-0264-5.
Hundal, Jasreet, Susanna Kiwala, Yang-Yang Feng, Connor J. Liu, Ramaswamy Govindan, William C. Chapman, Ravindra Uppaluri, et al. 2018.
“Accounting for Proximal Variants Improves Neoantigen Prediction.” Nature Genetics 51 (1): 175–79.
https://doi.org/10.1038/s41588-018-0283-9.
Hundal, Jasreet, Susanna Kiwala, Joshua McMichael, Christopher A. Miller, Huiming Xia, Alexander T. Wollam, Connor J. Liu, et al. 2020.
“pVACtools: A Computational Toolkit to Identify and Visualize Cancer Neoantigens.” Cancer Immunology Research 8 (3): 409–20.
https://doi.org/10.1158/2326-6066.cir-19-0401.
Jurtz, Vanessa, Sinu Paul, Massimo Andreatta, Paolo Marcatili, Bjoern Peters, and Morten Nielsen. 2017.
“NetMHCpan 4.0: Improved Peptide-MHC Class i Interaction Predictions Integrating Eluted Ligand and Peptide Binding Affinity Data,” June.
https://doi.org/10.1101/149518.
Keskin, Derin B., Annabelle J. Anandappa, Jing Sun, Itay Tirosh, Nathan D. Mathewson, Shuqiang Li, Giacomo Oliveira, et al. 2018.
“Neoantigen Vaccine Generates Intratumoral t Cell Responses in Phase Ib Glioblastoma Trial.” Nature 565 (7738): 234–39.
https://doi.org/10.1038/s41586-018-0792-9.
McLaren, William, Laurent Gil, Sarah E. Hunt, Harpreet Singh Riat, Graham R. S. Ritchie, Anja Thormann, Paul Flicek, and Fiona Cunningham. 2016.
“The Ensembl Variant Effect Predictor.” Genome Biology 17 (1).
https://doi.org/10.1186/s13059-016-0974-4.
Murphy, Charlie, and Olivier Elemento. 2016.
“AGFusion: Annotate and Visualize Gene Fusions,” October.
https://doi.org/10.1101/080903.
Nielsen, Morten, Claus Lundegaard, Ole Lund, and Can Keşmir. 2005.
“The Role of the Proteasome in Generating Cytotoxic t-Cell Epitopes: Insights Obtained from Improved Predictions of Proteasomal Cleavage.” Immunogenetics 57 (1-2): 33–41.
https://doi.org/10.1007/s00251-005-0781-7.
ODonnell, Timothy J., Alex Rubinsteyn, Maria Bonsack, Angelika B. Riemer, Uri Laserson, and Jeff Hammerbacher. 2018.
“MHCflurry: Open-Source Class i MHC Binding Affinity Prediction.” Cell Systems 7 (1): 129–132.e4.
https://doi.org/10.1016/j.cels.2018.05.014.
Ott, Patrick A., Zhuting Hu, Derin B. Keskin, Sachet A. Shukla, Jing Sun, David J. Bozym, Wandi Zhang, et al. 2017.
“An Immunogenic Personal Neoantigen Vaccine for Patients with Melanoma.” Nature 547 (7662): 217–21.
https://doi.org/10.1038/nature22991.
Rasmussen, Michael, Emilio Fenoy, Mikkel Harndahl, Anne Bregnballe Kristensen, Ida Kallehauge Nielsen, Morten Nielsen, and Søren Buus. 2016.
“Pan-Specific Prediction of PeptideMHC Class i Complex Stability, a Correlate of t Cell Immunogenicity.” The Journal of Immunology 197 (4): 1517–24.
https://doi.org/10.4049/jimmunol.1600582.
Richters, Megan M., Huiming Xia, Katie M. Campbell, William E. Gillanders, Obi L. Griffith, and Malachi Griffith. 2019.
“Best Practices for Bioinformatic Characterization of Neoantigens for Clinical Utility.” Genome Medicine 11 (1).
https://doi.org/10.1186/s13073-019-0666-2.
Rizvi, Naiyer A., Matthew D. Hellmann, Alexandra Snyder, Pia Kvistborg, Vladimir Makarov, Jonathan J. Havel, William Lee, et al. 2015.
“Mutational Landscape Determines Sensitivity to PD-1 Blockade in Nonsmall Cell Lung Cancer.” Science 348 (6230): 124–28.
https://doi.org/10.1126/science.aaa1348.
Rubinsteyn, Alexander, Isaac Hodes, Julia Kodysh, and Jeffrey Hammerbacher. 2017.
“Vaxrank: A Computational Tool for Designing Personalized Cancer Vaccines,” May.
https://doi.org/10.1101/142919.
Schumacher, Ton N., and Robert D. Schreiber. 2015.
“Neoantigens in Cancer Immunotherapy.” Science 348 (6230): 69–74.
https://doi.org/10.1126/science.aaa4971.
Shao, Xiaoshan M., Rohit Bhattacharya, Justin Huang, I. K. Ashok Sivakumar, Collin Tokheim, Lily Zheng, Dylan Hirsch, et al. 2020.
“High-Throughput Prediction of MHC Class i and II Neoantigens with MHCnuggets.” Cancer Immunology Research 8 (3): 396–408.
https://doi.org/10.1158/2326-6066.cir-19-0464.
Szolek, András, Benjamin Schubert, Christopher Mohr, Marc Sturm, Magdalena Feldhahn, and Oliver Kohlbacher. 2014.
“OptiType: Precision HLA Typing from Next-Generation Sequencing Data.” Bioinformatics 30 (23): 3310–16.
https://doi.org/10.1093/bioinformatics/btu548.
Uhrig, Sebastian, Julia Ellermann, Tatjana Walther, Pauline Burkhardt, Martina Fröhlich, Barbara Hutter, Umut H. Toprak, et al. 2021.
“Accurate and Efficient Detection of Gene Fusions from RNA Sequencing Data.” Genome Research 31 (3): 448–60.
https://doi.org/10.1101/gr.257246.119.
Vita, Randi, Swapnil Mahajan, James A Overton, Sandeep Kumar Dhanda, Sheridan Martini, Jason R Cantrell, Daniel K Wheeler, Alessandro Sette, and Bjoern Peters. 2018.
“The Immune Epitope Database (IEDB): 2018 Update.” Nucleic Acids Research 47 (D1): D339–43.
https://doi.org/10.1093/nar/gky1006.